The Use of Machine Learning to Improve Outcomes for Patients Undergoing Anesthesia
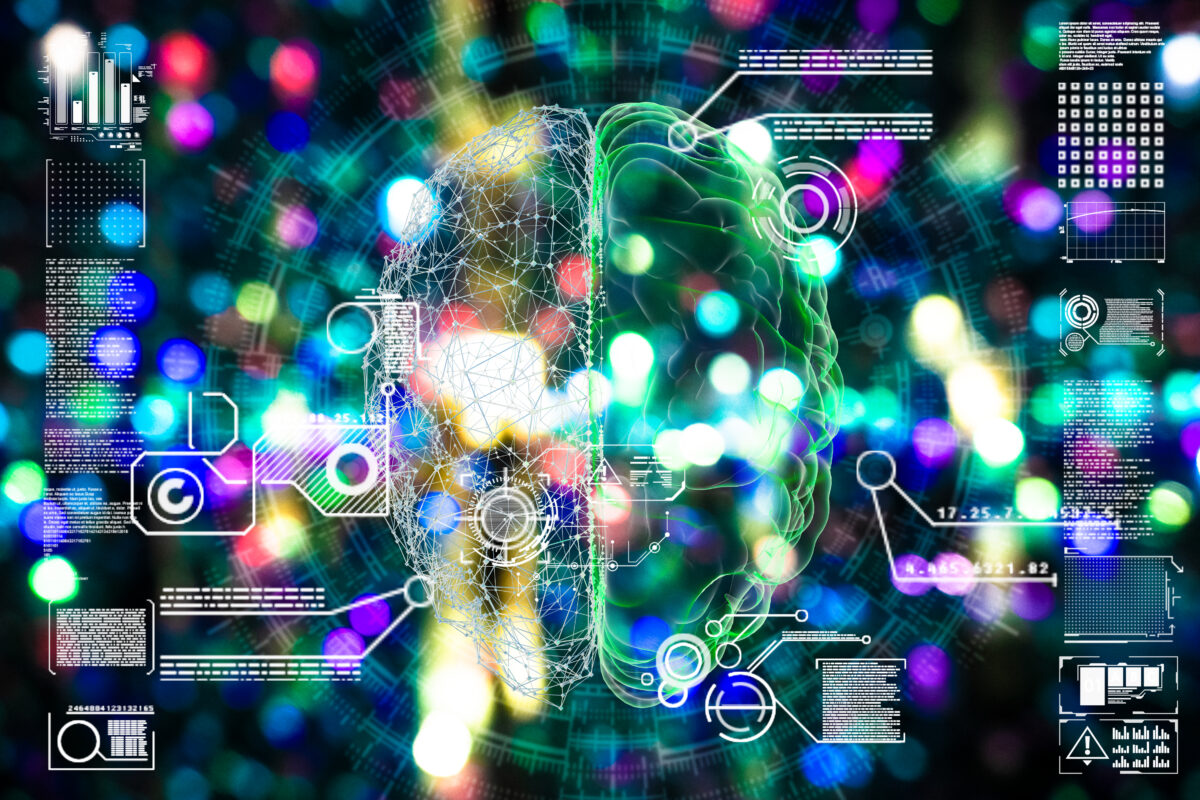
In the intricate realm of anesthesiology, patient safety and optimal outcomes remain paramount. As medical science evolves, the integration of machine learning (ML) into this field promises a transformative impact on patient care, particularly for those undergoing anesthesia. This integration is not just futuristic speculation; it is a present reality, reshaping the landscape of anesthesiology through enhanced predictive analytics, personalized care, and improved safety protocols.
Anesthesia is a critical component of surgical procedures, rendering patients insensible to pain by administering drugs that induce unconsciousness or sedation. However, it’s a delicate balance; too much can lead to complications, while too little can result in inadequate pain relief or awareness during surgery. The anesthesiologist’s role is to tailor the anesthesia to each patient’s needs, taking into account factors such as age, weight, medical history, and the type of surgery.
Machine learning, a subset of artificial intelligence, involves algorithms that learn from and make predictions or decisions based on data. In anesthesiology, ML can process vast amounts of data quickly, identifying patterns and insights that might elude human practitioners.
Predictive Analytics for Personalized Care
One of the most promising applications of ML in anesthesiology is predictive analytics. By analyzing data from past surgical cases, ML algorithms can predict how individual patients might respond to different anesthetic drugs or techniques. This personalized approach can help anesthesiologists make more informed decisions, reducing the risk of adverse reactions and improving overall patient outcomes.
Real-time Monitoring and Decision Support
During surgery, anesthesiologists must continuously monitor patients’ vital signs and adjust anesthesia levels accordingly. ML can enhance this process by providing real-time analysis of a patient’s physiological data. Advanced algorithms can detect subtle changes in vital signs and alert the anesthesiologist to potential issues before they become critical. This real-time decision support can be crucial in preventing complications and ensuring patient safety.
Enhanced Recovery and Postoperative Care
Machine learning can also play a role in postoperative care. By analyzing data on pain levels, medication usage, and recovery progress, ML algorithms can help tailor pain management and rehabilitation plans to each patient’s needs. This can lead to faster recoveries and better long-term outcomes.
Training and Simulation
Machine learning can also contribute to the education and training of future anesthesiologists. Through advanced simulation models that replicate real-life scenarios, ML can provide trainees with realistic experiences, helping them develop the skills and judgment needed to manage complex cases.
Despite its potential, the integration of machine learning into anesthesiology is not without challenges. Data privacy and security are paramount concerns, as patient data is highly sensitive. Additionally, there is the risk of algorithmic bias if the data used to train ML models is not representative of the diverse patient population.
Furthermore, there are ethical considerations regarding the reliance on algorithms for decision-making. The role of the anesthesiologist cannot be fully replaced by technology; human judgment and experience remain crucial, especially in unpredictable or complex cases.
The integration of machine learning into anesthesiology represents a significant leap forward in patient care. By leveraging predictive analytics, real-time monitoring, and personalized care plans, ML has the potential to improve outcomes for patients undergoing anesthesia significantly. As this technology continues to evolve, it promises to enhance the precision, safety, and effectiveness of anesthetic practices.
However, it is essential to navigate this advancement with caution, ensuring that ethical considerations and patient safety remain at the forefront. The future of anesthesiology, augmented by machine learning, holds immense promise, but it must be approached with a balanced understanding of both its capabilities and limitations.